DTC Announces Release of CCPP Single-Column Model v7.0.1 with Enhanced Capabilities and Documentation
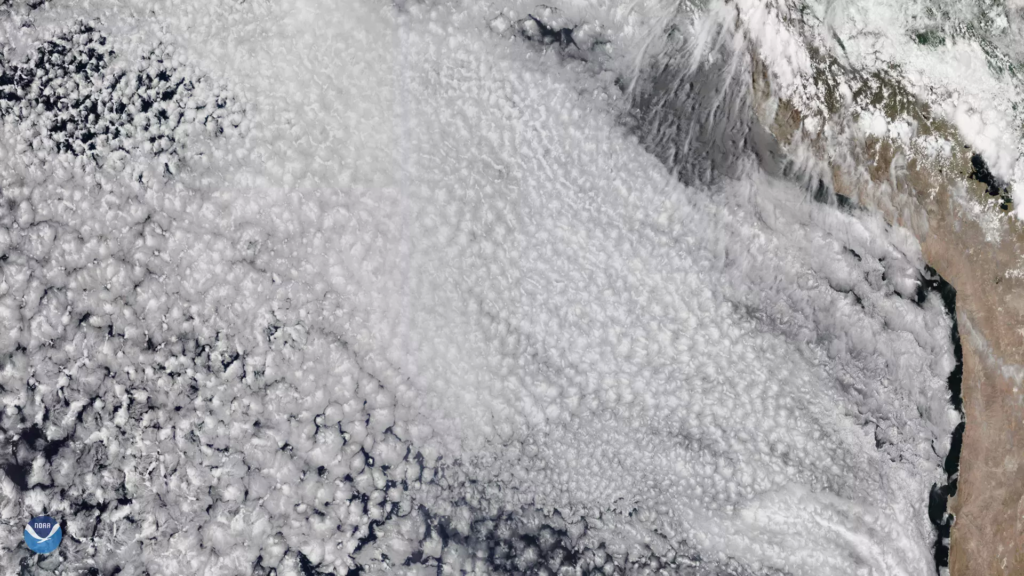
Modeling is an essential component of NOAA’s services and capabilities value chain. The NOAA Modeling Strategy (2024) provides a 10-year framework to advance cutting-edge research, development, and operational implementation activities for all modeling that is foundational to NOAA’s science and service missions. This strategy positions NOAA to be the global leader in Earth System Modeling as set forth under the principles of the Earth Prediction Innovation Center (EPIC) and the Unified Forecast System (UFS) to implement open-source and community modeling approaches to increase collaboration with the broader community where possible.
New Idealized, Regional Tropical Cyclone Test Case Added to UFS Weather Model

Modeling is an essential component of NOAA’s services and capabilities value chain. The NOAA Modeling Strategy (2024) provides a 10-year framework to advance cutting-edge research, development, and operational implementation activities for all modeling that is foundational to NOAA’s science and service missions. This strategy positions NOAA to be the global leader in Earth System Modeling as set forth under the principles of the Earth Prediction Innovation Center (EPIC) and the Unified Forecast System (UFS) to implement open-source and community modeling approaches to increase collaboration with the broader community where possible.
Community Modeling on Community Platforms – One member’s perspective on the Unified Forecast System
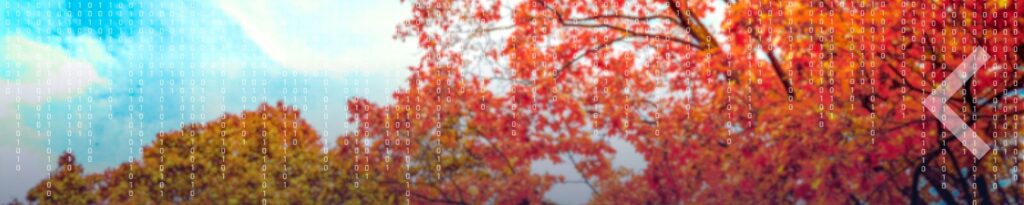
Doing new and interesting science with the Unified Forecast System (UFS), or any numerical model, requires completing a similar series of basic steps. These steps are easy to define but can be challenging to execute, especially for platforms and environments that are very different from where the model was developed. Working in collaboration with our partners at NOAA Earth Prediction Innovation Center (EPIC) and Environmental Modeling Center (EMC), researchers at George Mason University have succeeded in implementing both the UFS and the EMC global-workflow on multiple community platforms. Our team is now in full production, making runs and analyzing data in support of the Seasonal Forecast System development effort.
NWS launches Warn-on-Forecast System Demonstration Project
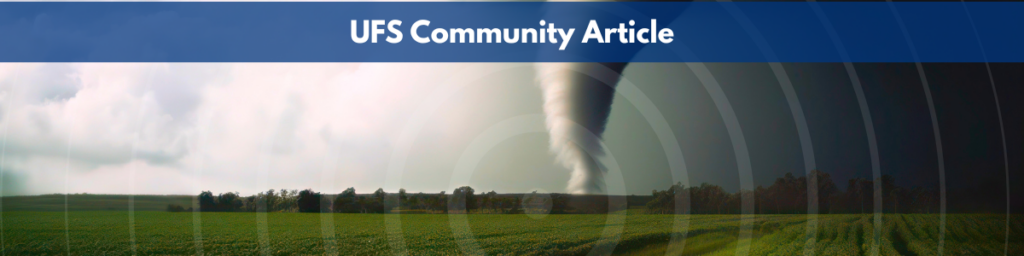
Doing new and interesting science with the Unified Forecast System (UFS), or any numerical model, requires completing a similar series of basic steps. These steps are easy to define but can be challenging to execute, especially for platforms and environments that are very different from where the model was developed. Working in collaboration with our partners at NOAA Earth Prediction Innovation Center (EPIC) and Environmental Modeling Center (EMC), researchers at George Mason University have succeeded in implementing both the UFS and the EMC global-workflow on multiple community platforms. Our team is now in full production, making runs and analyzing data in support of the Seasonal Forecast System development effort.
Forecasting Made Accessible: AI Weather Models on Google Colab
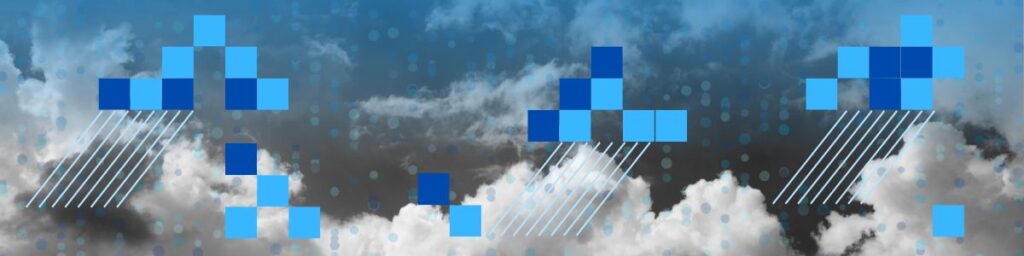
Dr. Jacob Radford (GSL/CIRA) has done an outstanding job creating a comprehensive series of Google Colab notebooks that enable users to run Artificial Intelligence (AI) Weather Prediction (AIWP) models using free resources. The flagship notebook, Running_AIWP.ipynb – Colab, provides an in-depth overview of AIWP models, including how to assess forecast accuracy and the overall run time of these models. Building on the foundational work of the European Centre for Medium-Range Weather Forecasts (ECMWF)’s ai-models Python package, Dr. Radford meticulously guides users through various models such as FourcastNet, Pangu-weather, Graphcast, and Aurora, offering an interactive tutorial to execute them on Google Colab. With an estimated run time of 70 minutes, this resource is a testament to Dr. Radford’s dedication to advancing innovative approaches in scalable AIWP.
HAFS Forecasts Contribute to More Effective Emergency Response
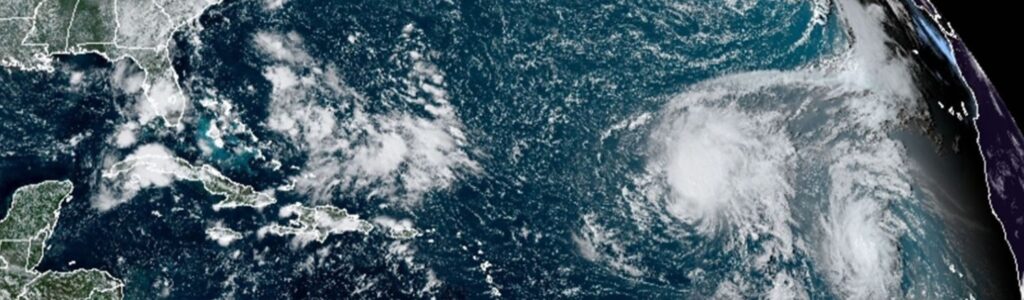
Modeling is an essential component of NOAA’s services and capabilities value chain. The NOAA Modeling Strategy (2024) provides a 10-year framework to advance cutting-edge research, development, and operational implementation activities for all modeling that is foundational to NOAA’s science and service missions. This strategy positions NOAA to be the global leader in Earth System Modeling as set forth under the principles of the Earth Prediction Innovation Center (EPIC) and the Unified Forecast System (UFS) to implement open-source and community modeling approaches to increase collaboration with the broader community where possible.
Artificial Intelligence in Weather Modeling Workshop
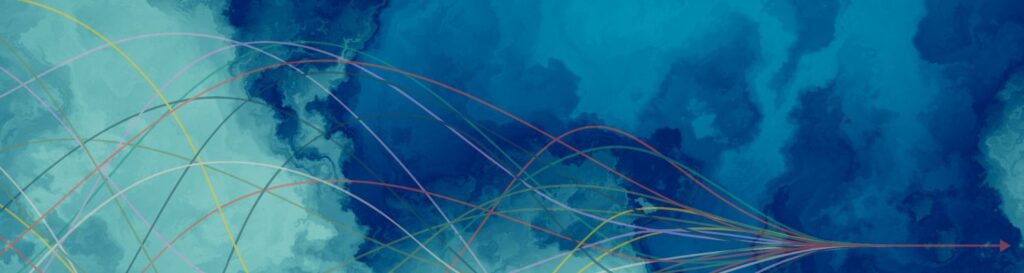
The Earth Prediction Innovation Center (EPIC) invites you to a one-day Artificial Intelligence (AI) in Weather Modeling Workshop on June 27, 2025. This virtual event will explore cutting-edge AI applications in numerical weather prediction (NWP), featuring hands-on demonstrations of GraphCast, FourCastNetv2, and PanguWeather, as well as an introduction to the European Centre for Medium-Range Weather Forecasts (ECMWF) Anemoi Core framework.
UFS Short-Range Weather (SRW) Application v3.0.0 Release
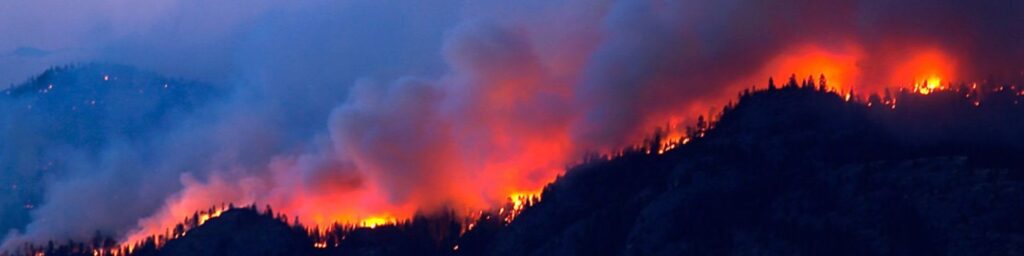
The Earth Prediction Innovation Center (EPIC) and the Unified Forecast System (UFS) community are proud to announce the public release of the Short-Range Weather (SRW) Application (App) v3.0.0, more information is available via the EPIC SRW Get Code page. This release is an update to the v2.2.0 release from October 2023 and incorporates a number of changes currently available in the SRW App develop branch, including significant enhancements to the SRW App’s ability to to simulate smoke, dust, and two-way coupled fire-atmosphere interactions.
CADRE-EPIC Data Assimilation Training
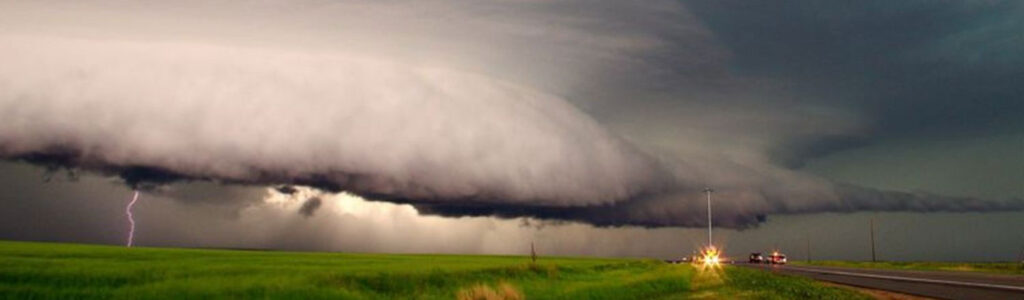
The Consortium for Advanced Data Assimilation Research and Education (CADRE), in partnership with the NOAA Earth Prediction Innovation Center (EPIC), will host a data assimilation training event on June 4-6, 2025, at the Colorado State University.
EPIC’s Code Management Team Implements Automated Jenkins Pipeline for CI/CD in NOAA PSL Stochastic Physics Repository
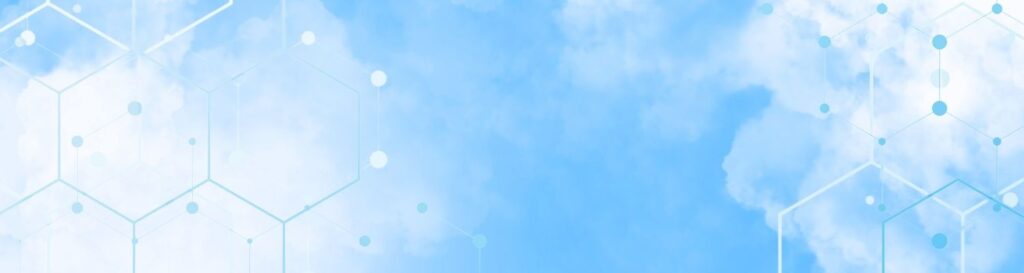
The EPIC Code Management team has successfully developed and integrated a new automated Jenkins pipeline for unit testing code changes in the NOAA Physical Sciences Laboratory (PSL) Stochastic Physics repository. This pipeline streamlines the software development lifecycle by enabling Continuous Integration (CI), Continuous Delivery (CD), and Continuous Deployment (CD) through an efficient, automated process. By simply adding a GitHub label to a pull request, EPIC Code Managers can now trigger unit tests and seamlessly progress through the CI/CD pipeline.